题目:Forward-Selected Panel Data Approach for Program Evaluation
主讲人:史震涛,香港中文大学
时间:2019年9月27日,15:00-16:15
地点:暨南大学中惠楼106B室
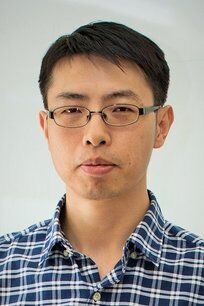
主讲人简介:
He joined the Chinese University of Hong Kong in 2014 as Assistant Professor in the Department of Economics. He specializes in econometric theory, in particular estimation and inference of machine learning methods in economic applications. His English papers have been published in the journals of Econometrica, Journal of Econometrics, Journal of Applied Econometrics, Econometric Reviews and so on. Before working in Hong Kong, he obtained his Ph.D. from Yale University in 2014, M.A. from Peking University in 2008, and B.A. (finance) from Zhejiang University in 2005.
Abstract:
Policy evaluation is central to economic data analysis, but economists mostly work with observational data in view of limited opportunities to carry out controlled experiments. In the potential outcome framework, the panel data approach (Hsiao, Ching and Wan, 2012) constructs the counterfactual by exploiting the correlation between cross-sectional units in panel data. The choice of cross-sectional control units, a key step in their implementation, is nevertheless unresolved in data-rich environment when many possible controls are at the researcher's disposal. We propose the forward selection method to choose control units, and establish validity of post-selection inference. Our asymptotic framework allows the number of possible controls to grow much faster than the time dimension. The easy-to-implement algorithms and their theoretical guarantee extend the panel data approach to big data settings. Monte Carlo simulations are conducted to demonstrate the finite sample performance of the proposed method. Two empirical examples illustrate the usefulness of our procedure when many controls are available in real-world applications.